In today's data-driven world, businesses constantly seek ways to gain insights, make informed decisions, and stay ahead of the competition.
One powerful tool that has emerged to address these needs is predictive analytics. This comprehensive guide will explore predictive analytics, its significance for businesses, practical examples, recommended tools, and how to leverage it to drive success in your organization.
What is Predictive Analytics?
Predictive analytics involves using historical data, statistical algorithms, and machine learning techniques to identify patterns and predict future outcomes. By analyzing past behaviors and trends, predictive analytics enables organizations to forecast future events, anticipate customer needs, optimize processes, and mitigate risks.
At its core, predictive analytics goes beyond traditional descriptive and diagnostic analytics by focusing on what may happen in the future rather than just explaining what has happened in the past. It empowers businesses to make proactive decisions and take strategic actions based on data-driven insights.
Predictive Analytics: Meaning and Definition
Predictive analytics is extracting valuable insights from data to forecast future trends, behaviours, and events. It involves the application of statistical models and algorithms to analyze historical data, identify patterns, and make predictions about future outcomes with a high degree of accuracy.
Predictive analytics enables organizations to leverage data-driven insights to anticipate changes in market dynamics, customer preferences, and business conditions. By harnessing the power of predictive analytics, businesses can optimize operations, enhance decision-making processes, and gain a competitive edge in their respective industries.
Predictive Analytics Examples
To illustrate the practical applications of predictive analytics, let's consider a few examples across different industries:
Retail: A retail chain utilizes predictive analytics to forecast customer demand for specific products based on historical sales data, seasonality trends, and demographic information. The retailer can optimize inventory levels, minimize stockouts, and improve overall sales performance by accurately predicting demand.
Finance: A financial institution employs predictive analytics to assess credit risk and determine the likelihood of loan default. By analyzing factors such as credit history, income levels, and payment behavior, the institution can make more informed lending decisions, mitigate risks, and safeguard its financial assets.
Healthcare: A healthcare provider leverages predictive analytics to identify patients at risk of developing chronic conditions such as diabetes or heart disease. By analyzing patient demographics, medical history, and lifestyle factors, healthcare professionals can intervene early, implement preventive measures, and improve patient outcomes.
Marketing: A marketing agency uses predictive analytics to segment customers based on purchase behavior, preferences, and engagement patterns. By identifying high-value segments and predicting future buying behaviors, marketers can tailor marketing campaigns, personalize messaging, and optimize marketing spend for maximum ROI.
Read this for a practical example.
Tools for Predictive Analytics
Several tools and software platforms are available to support predictive analytics initiatives in businesses of all sizes. Some popular tools include:
- IBM Watson Studio: Offers a comprehensive suite of tools for data preparation, model development, and deployment of predictive analytics solutions.
- SAS Predictive Analytics: Provides advanced analytics capabilities, including machine learning algorithms, forecasting models, and data visualization tools.
- Microsoft Azure Machine Learning: Enables users to build, train, and deploy machine learning models at scale, with integrated support for predictive analytics workflows.
- Google Cloud AI Platform: Offers a range of machine learning services and tools for building predictive models, analyzing data, and generating insights.
These tools provide businesses with the necessary infrastructure and capabilities to conduct predictive analytics projects effectively, from data preprocessing and model training to deployment and monitoring.
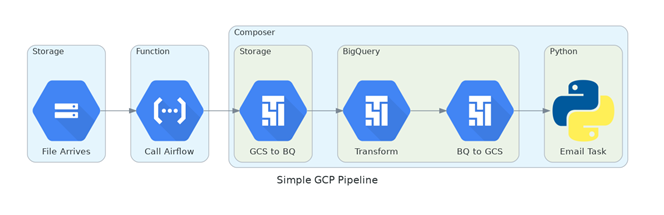
Analytics represents a transformative force in today's business landscape, enabling organizations to anticipate trends, make informed decisions, and drive sustainable growth.
By harnessing the power of predictive analytics, businesses can unlock valuable insights from their data, optimize operations, and gain a competitive advantage in their respective markets.
If you're ready to explore the potential of predictive analytics for your business or have any questions about implementing predictive analytics solutions, feel free to contact us. Our team of experts is here to help you harness the power of data-driven insights and propel your business forward.