User experience is more important than ever to success for a profitable online business. Customer Experience determines how customers speak or write about your company. What can be done to make sure it is positive? How can you create repeatable and personalized experiences?
In this article I'm focusessing on main aspects for improving and automatizing user experience, how and where are the best spot to show recommendations.
Status Quo
In my personal experience, I noticed in most of the times, even in enterprise level e-commerce, an almost surreal way of managing products placement as if it were a physical point of sale.
Weekly updates in product placements in Homepage, used as a virtual "showcase", communicated to their e-commerce manager, and using of promotions and sponsoring as the only sales drivers.
40% of online merchandising becomes obsolete or does not justify its presence because it doesn't make any sales.
It's quite simple for insiders solve merchandising saturation and implement personalization, due to more and more easier tools. Market is continually evolving allowing less invasive and easier implementations, avoiding onerous ingegnerization costs.
What are machine learning recommendations?
It's an algorithm that suggests (or recommend) products tailored on specific customer preferences, on his navigation history and purchasing behaviors using statistical forecasts, it suggests products most likely to be purchased.
Substantial difference, between one algorithm and another, is given by the number of features and the data collected, to allow a "more pertinent" prediction as relevant as possible for customers.
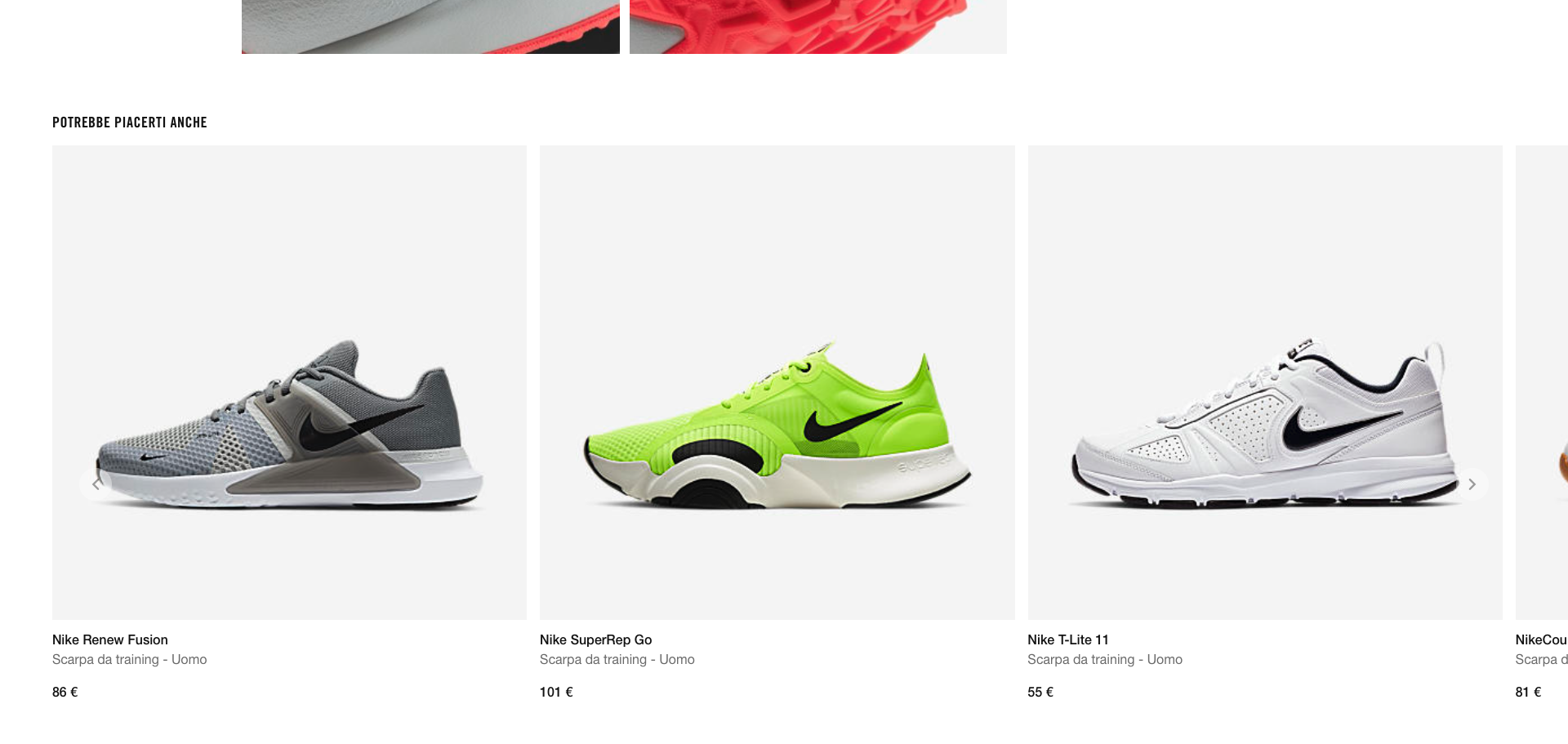
How do help improving shopping experience?
Basically it predicts (using different accurancy level) most commonly purchased products, according to specific parameters, provide indications suited to each user (also to those has not purchased yet) and much more relevant to those who have purchased.
Imagine that during a your returning navigation on your preferred e-commerce, the related products or those shown after a search query, are relevant to your interests which are similar to many other users.
For example: if you try browse Nike e-commerce, after viewing only 3 products on their store, they are able to suggest a more pertinent recommendation then others. Try to add one in the shopping cart and go to cart page, you'll notice a "Suggested" section, below the page. These recommendations are proposed to a customer cluster (where you're currently in) more likely to buy or those I seen previously (it depends on the machine learning algorithm applyed).

In my case, It would have been more useful if they had offered me socks or shorts in a color relevant to what I'm buying, at a discounted price (😂).
Where should you embed recommendations
The locations for embedding recommendations are generally those were heatmaps are medium hot spots.
Some of most common positions are:
- Homepage
- Search results
- Product
- Cart
- Error pages
Another most common useful section is autocomplete in the search bar. I wrote a work where you'll find all results after implementation, I think you should find it useful.
Homepage
It's crucial for product recommendations, as reported in Baymard Institute research where 25% of test visitors to scroll down and up the homepage to quickly evaluate the site’s products.
Great commerce experiences rely on effective customer segmentation based on behaviors, demographics, and interests.
Search results
The ideal search page incorporates recommendations that show what other customers viewed and purchased during their browsing, starting from this point to purchase thank you page.
By the way, in this page the filters are so much important too: shown in the left sidebar and only those adherent to search results.
Product
Set up recommended up-sell to show products with similar or higher prices (or both) to increase AOV. This stimulates customers' interest in continuing search, and at the same time allows you to promote a several different related products.
Some years ago Amazon revealed that 35% of its revenue in the product page are made by cross-selling and up-selling sections.
Cart
This page is the perfect moment for up-selling offering complementary items to complete a customer’s order. Remind your customer about the most relevant products browsed.
Up to 25% of customers who click on these recommendations order it.
Error page
When you’re pleasantly browsing your favourite e-commerce, finding a product you like and being hit with a 404 message is a shame!
And take it down your willing to purchase.
Instead of redirecting to other pages on the site or simply showing an error message, why not turn the inconvenience into an opportunity? Use error pages to display:
- Best sellers: keep your customers engaged with most hot products;
- Based on browsing history: display items based on the customer’s product breadcrumb;
- Related/Similar Product: suggest your customer other items similar to what they were searching.
These are some practical examples, applicable today with no technical efforts due most of the recommendation tools are implemented through modules, tracking scripts and / or html markup, where the suggested products are "injected" in real time.
Note – For Advanced Use
Depending on a particular browsing behavior, these contents should be filtered with specific rules based on price or merchandising needs.
Each recommended content, you can also apply an additional subdivision strategy, using information gathered previously about their AOV (Average Order Value) or what products they have recently purchased available:
- suggesting in Cross Selling section, to a defined cluster (Champion or Frequent Buyer, for example) and offering them all those products with an higher price, because it's more in line with their spending history;
- suggesting, in search results page (taking advantage of the mispelling too), more relevant products to a specific cluster that is searching somthing useing a generic keyword, for example: white shoes, red bag, etc.;
- in Cart page, suggest complementary products, most commonly purchased by VIP segment, compared to those they're purchasing.
About Sales Boost
I will write some selected works where there has been a major increase in overall e-commerce sales, with x2, x3 or even x5.
In Work page, you'll find those I selected among the most challenging, starting from oldests, I am continuing updating that, at least once a week.
Subscribe to the newsletter to be notified!
Results implementing recommendations
Here, I increased sales for all funnels sourcing from search by 300%. This means that the business revenue stream has undergone an increase, not by 300%, but a proportional increase to the conversion that occurred from the sessions that started with the use of the search bar.
Example: if 10% of users using search bar for finding a product that it'll be purchased, and the starting conversion rate is 3%, increase it by x2, it means double these sales.
If recommendations were also applied to other on-platform funnels, it would be possible get an exponential sales increase curve.
The revenue stream drastically boosting of marketing automations is applied with recommendations.